Sorry, page not found
Sorry, page not found
The link you followed may be broken, or the page may have been removed. You may search
for whatever you’re looking for here.
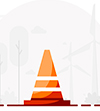
More from Jio
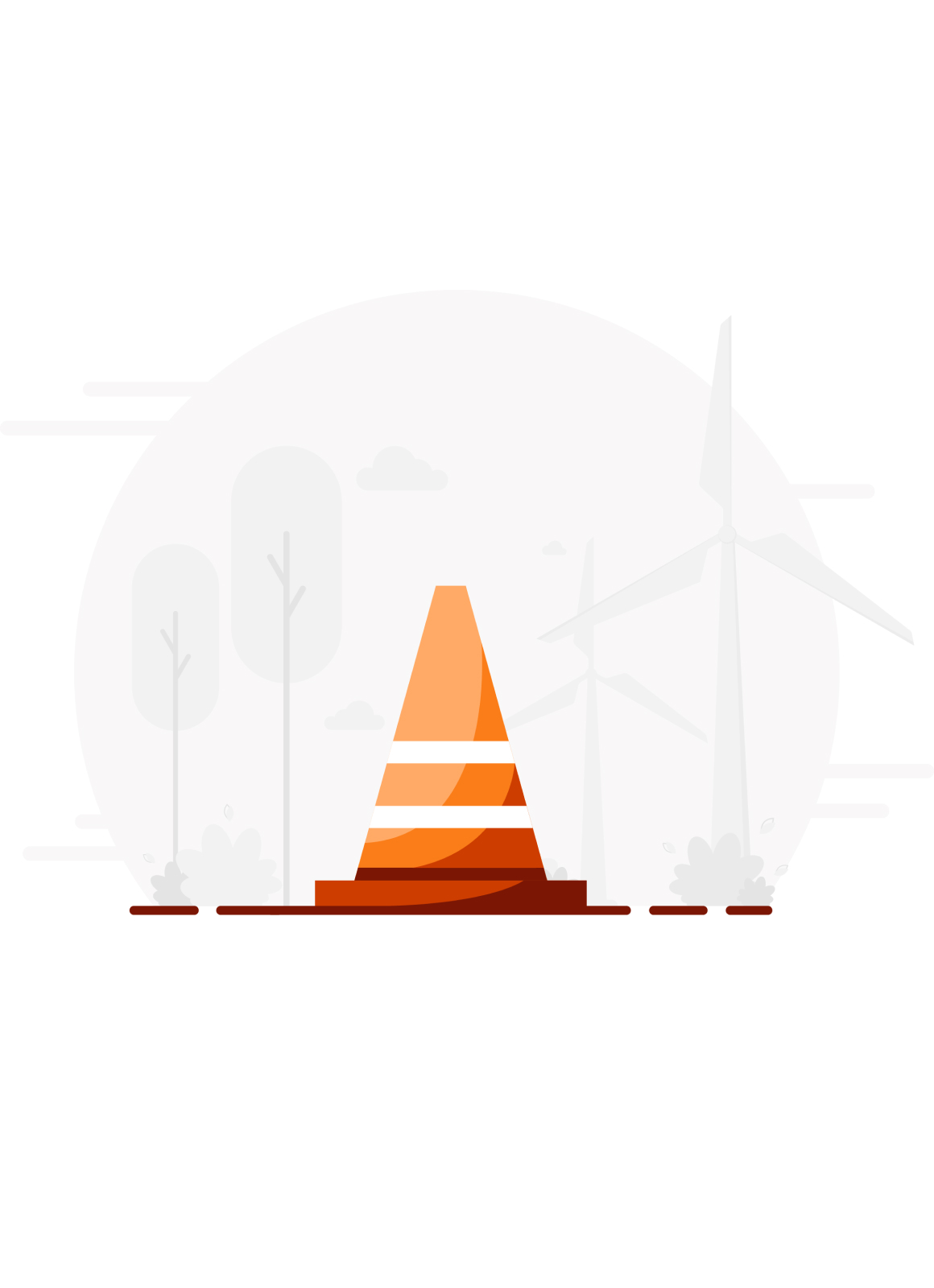